IITK - Rice Strategic Collaboration Award Winners

Carbonatites and World's Rare Earth Element Reserves: A Combined Experimental and Geochemical Study
A global initiative to transition to zero-carbon energy sources will require rare earth elements (REEs) and tungsten (W) for several major renewable energy technologies. Most of the world’s REEs come from carbonatites, carbonate-dominated igneous rocks (crystallized product of magma or lava), and their alteration products. However, no unified model explains all features of carbonatite-associated REE deposits, strongly impairing exploration required to secure future supply. In this collaborative project, we seek to combine experimental and geochemical approaches to understand the processes of REE and W concentrations in carbonatites. We will study natural samples from Amba Dongar and Kamthai carbonatite complexes of India. Guided by the natural compositions, we will also conduct laboratory experiments to determine pathways of REE concentrations in carbonatite and associated phases. A specific goal will be to better constrain partitioning of REEs and other key elements between carbonate, silicate, and oxide phases in carbonatites.
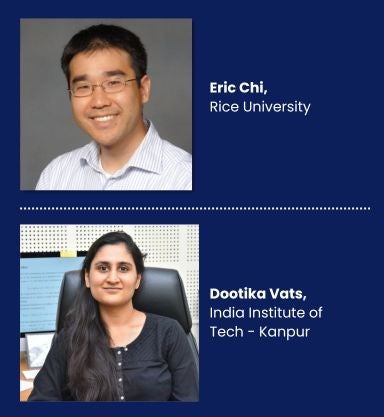
Uncertainty Quantification: Leveraging Synergy between Optimization and Sampling
Artificial Intelligence (AI) and machine learning (ML) have made amazing progress towards automating tasks and making predictions. As we aim to automate more nuanced tasks and make predictions in more complicated scenarios, the need for quantifying uncertainty around models is critical. In fields such as finance, engineering, climate science, and healthcare, accurate predictions and reliable decision-making under uncertainty are essential. This collaboration combines expertise from two complementary frameworks for training AI and ML models which historically have been developed in parallel with relatively little interaction: sampling and optimization. Sampling traverses the solution space for viable solutions; optimization seeks the best solution within a set of constraints. Designing algorithms from a joint perspective will enable exploring solution spaces more comprehensively and efficiently towards learning models and quantifying their uncertainty. An IIT-K student will be hosted at Rice to catalyze the research and pilot regular visits between the PI’s groups.
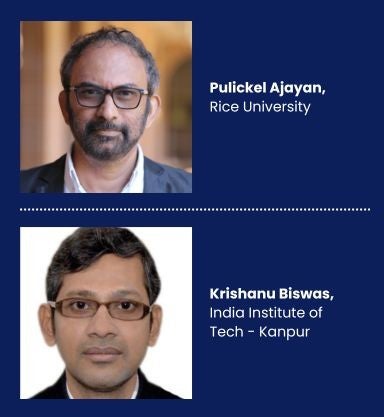
Development of High Entropy Alloy nanoparticles for Hydrogen Production from Sustainable Resources
Hydrogen (H2), being the green fuel with one of the highest gravimetric energy densities, can potentially replace the non-renewable fossil fuels and has ubiquitous usage in the chemical and petroleum industries. We propose to modulate the surface electronic structure, microstates, and chemical properties of nanoengineered high entropy alloys (HEAs) composed of earth-abundant TMs for sustainable production of H2 from three abundant resources viz. water (H2O), methane (CH4), and hydrogen sulfide (H2S). The chemistry of the catalysts will be selected and fine-tuned by DFT-ML calculations. The electronically tuned materials thus will exhibit better electron transfer in the active sites and an optimal adsorbate (in this case H*/CH4* etc.) binding energy. We envision that the synergistic tuning of structural and chemical properties of the HEAs will lead to a class of easily scalable inexpensive catalysts that will be efficient and durable to produce hydrogen from multiple resources for industrial adaptation.
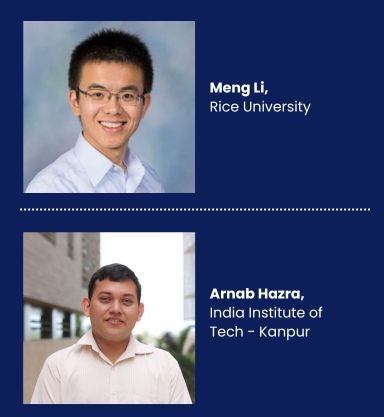
Development of High Entropy Alloy nanoparticles for Hydrogen Assessing Climate Change Impacts on Marine Ecosystems: Bayesian Spatiotemporal Modeling for Predicting Sea Surface Temperature Dynamics and Identifying Hotspots in the Red Sea
Advancing our understanding of climate change necessitates sophisticated modeling approaches. This project proposes a novel Bayesian spatiotemporal model to dissect sea surface temperature changes in the Red Sea, a vital indicator of climate change. The model introduces a nuanced approach to capture the rate of change, recognizing the complexities that arise when tracking dynamic spatiotemporal patterns. Furthermore, our model addresses challenges in hotspot detection, particularly rate-based hotspots and shape constraints, by employing flexible statistical techniques for the accurate identification of hotspots with principled uncertainty quantification that is currently lacking. Leveraging the combined expertise in complex data modeling with theoretical guarantees and environmental research, this project will advance big data analytics in climate change studies. The developed spatiotemporal models will facilitate enhanced climate change assessment, enabling more informed policymaking for marine ecosystem conservation. Though our focus is the Red Sea, our methodologies are applicable to other spatiotemporal datasets, leading to broad impacts.
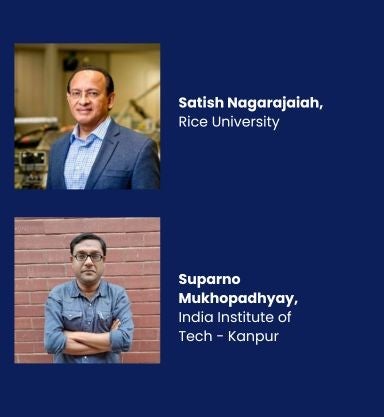
Structural Health Monitoring of Wind Turbine Blades: Optimal Sensor Location, Parameter Identification and Damage Assessment under Environmental Variability with Fusion of Strain- Acceleration Data
This project focuses on vibration-based monitoring of wind turbine blades, necessary for the safe and uninterrupted operations of wind turbines. The proposed research involves identification of dynamic models, including a high-fidelity FE model, of a blade using benchmark test data. A study will be then performed to determine damage sensitive features most suitable for detecting presence and location of damages in the blade. Nonlinear filtering will be used to detect the extent of damages. The methods will be developed in a framework: (i) based on strain-acceleration data fusion for improved accuracy, and (ii) to factor out effects of temperature variability on damage assessment. An optimal sensor location strategy will also be developed to minimize uncertainty in damage detection. The project will result in a robust and reliable health assessment technique for wind turbine blades, and will pave the way for further research and applications such as non-contact and operational monitoring.”
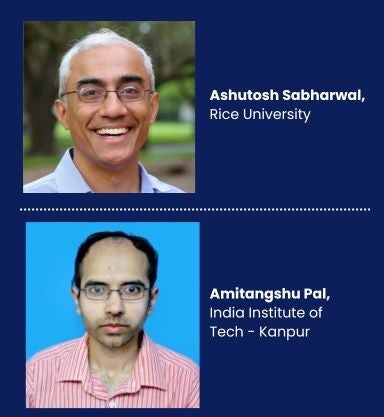
A Tracking System for Trapped Victims in Emergency Rescue Operations
Rescue operations in case of building collapse sometimes take several hours to days; in fact, even after that the rescue personnel often cannot find the victim's bodies that are buried under the debris. Very often the responders need to cut the debris extensively to find if anyone is still trapped inside and if yes, the location for the trapped individual. Therefore, tracking the positions of trapped victims can provide critical information to the rescue personnel that can potentially save lives. We will develop a radar-assisted rescue-aid system that will aim to locate buried individuals in a rubble. To achieve the envisioned system, we will (i) significantly improve the angular resolution of radars using a combination of physics-inspired machine learning algorithms, and (ii) develop new algorithms to undo the multipath effects of propagation due to rubble. The algorithms will be quantified using a combination of simulations and targeted experiments
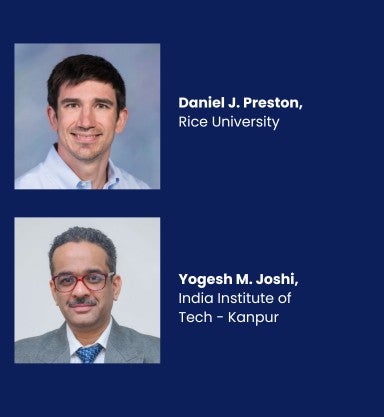
Edible Metamaterials via 3D Printing for Enhanced Food Properties
3D printing of food is an emerging manufacturing technique that has opened new avenues for advancements in personalized nutrition, texture, and taste. However, existing food 3D-printing research is often limited to empirically trying new material compositions and printing techniques to change food properties without an underlying understanding of the food rheology and mechanics. The goal of this project is to systematically characterize the rheological properties of a diverse array of food inks suitable for 3D food printing, along with the resultant food structure and mechanics, in order to achieve edible metamaterials with enhanced and tunable food properties in comparison to conventional methods. This project supports the collaborative efforts of investigators with expertise in rheology, materials science, and mechanics, including travel to international conferences and submission of proposals focused on food sustainability and health.
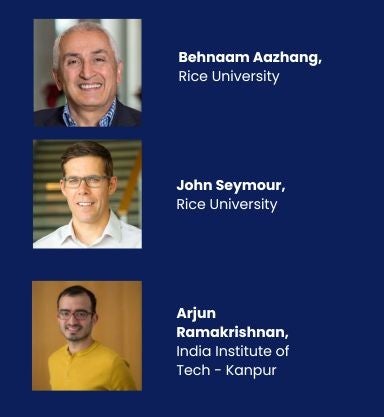
Information models of the ACC to drive high-density, multi-region depth array based mapping during a decision task
Anterior Cingulate Cortex (ACC), part of our frontal lobe, is hypothesized to be critical in flexibly regulating behavior in a dynamically changing environment. ACC subregions have been implicated in monitoring uncertainty, learning and decision making. Given the diversity of functions regarding the ACC, we will leverage a novel recording tool and novel computational method to greatly improve the information available to researchers in the non-human primate ACC. We will demonstrate this by generating virtual information models of these brain regions and find the optimal placement of electrodes. We will target ACC subregions and nearby prefrontal regions as defined by fMRI pilot study in humans. This seed funding will strengthen neurotechnology research efforts across Rice and IIT-Kanpur and combine expertise in primate neuroscience, neurotechnology, and information theory. We anticipate broad impact in high precision computational mapping of specific brain regions involved in decision making under uncertainty, which will not only have direct translatability for clinical applications, but also significantly advance our understanding of the neural mechanisms of the cognitive processes under consideration.